For more than two decades, customer relationship management (CRM) and enterprise resource planning (ERP) software have been defined by manual entry and high-touch data processes. Since then, businesses have made strides in automating many manual transactions through various means but have largely reached a plateau. Our 2023 survey on business trends found that 9 out of 10 workers hope to use AI to reduce these kinds of repetitive tasks from their jobs.1
Supply chains have been a prime area for the application of AI, due to the vast amounts of critical business data and processes involved. Supply chains have evolved over the years, with emerging technologies and innovations that enable businesses to optimize their operations, reduce costs, and improve customer satisfaction. Yet, while statistical models have been used in processes such as inventory management, forecasting, production planning, and scheduling, there hasn’t been a significant shift in the industry beyond improving algorithms. Learning hasn’t been applied to make supply chain processes intelligent and self-regulating. The next generation of AI will transform the industry by making it more agile, efficient, and responsive to changes.
In March, Microsoft announced Microsoft Dynamics 365 Copilot, introducing the world’s first AI copilot for ERP and CRM applications. With the next generation of AI capabilities in Dynamics 365 Copilot, those high-touch, laborious processes can be transformed with interactive AI-powered assistance.
With Copilot, you can further unlock the potential of ERP by bringing together data and AI to reduce time spent on unfulfilling tasks and accelerate the speed of execution and business outcomes.
We are excited about bringing next-generation AI to virtually every business function, and especially so about the opportunities AI has within the Microsoft Supply Chain Platform. In this post, we look at AI in supply chain management (SCM), both its development and current state, and we share our view of next-generation AI in SCM.
Learn more: Introducing Microsoft Dynamics 365 Copilot, bringing next-generation AI to every line of business
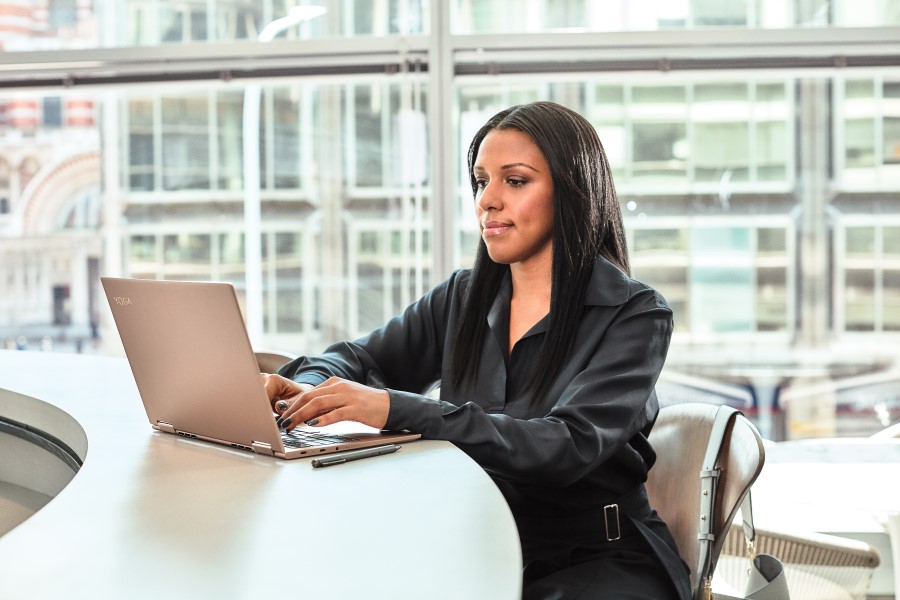
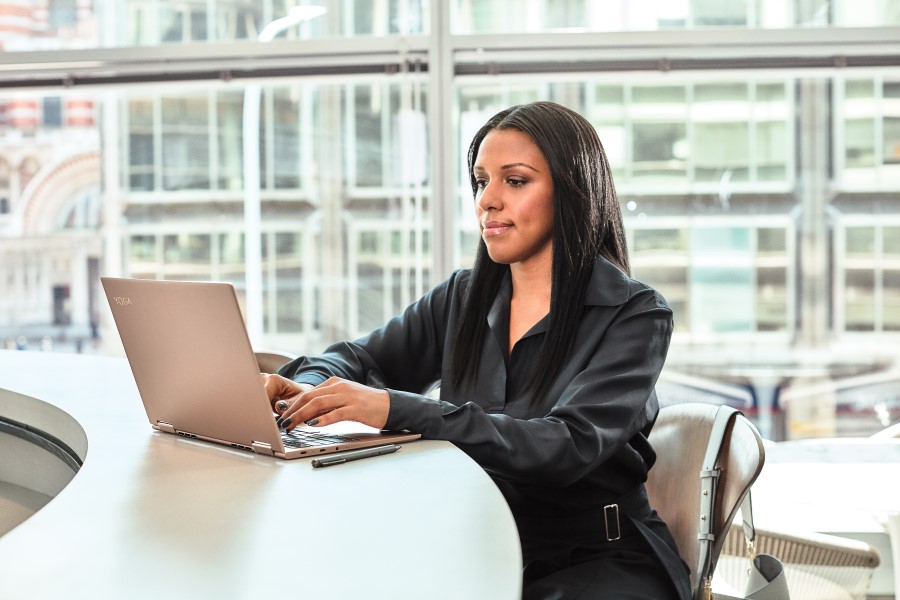
Microsoft Supply Chain Center
Redefine what’s possible with the power of AI.
Industry 4.0 is AI
The Fourth Industrial Revolution opens doors that further transform how we work and what we focus on during the workday. We have come a long way from the industrial plant floor automation of the 1960s to the intelligent era of supply chain digital twins of the 2020s. We now have significant technological advances that can transform traditional supply chains into next-generation cognitive supply chains. These cognitive supply chains can proactively predict and self-correct disruptions, trigger replanning, and provide intelligent recommendations. Thus, enabling humans and AI to work together to quickly respond, in real time, to changing environments.
In the age of AI, tools like artificial neural networks and machine learning provide the means to automate personal workflows and processes. Still, low-code applications, natural language processing, and generative AI will not replace human innovation. It will, on the other hand, increase and expand our expertise and ability. This is why at Microsoft, we believe that AI is going to define new ways for humans to amplify their impact, their capability, and their unique potential.
The brief history of AI in supply chain management processes
The first application of AI in SCM is a so-called expert system known as the inventory management assistant (IMA). IMA was designed in 1986 to improve the replenishment of spare parts and reduce safety stock for the US Air Force.2 From there, the 1990s saw a broad resurgence of interest in the decades-old concept of AI. As a result, AI became commercially available in SCM applications on a limited basis during this time.
In the 2000s, computing power continued to increase as hardware costs declined rapidly, making the investment in AI affordable. However, AI’s widespread adoption in SCM really took off in the 2010s with the rise of the Industrial Internet of Things (IIoT) and the associated acceleration in digital transformation. Together, these factors led to an explosion in the amount of data generated by supply chain processes, marking the beginning of big data in the supply chain.
Outside the supply chain, machine learning algorithms matured and refined into efficient and almost standard-like features, such as the Netflix recommendation engine. At the same time, SCM use cases were taking shape and beginning to deliver value. The first applications of machine learning came in the areas of demand forecasting using regression models to achieve high forecast accuracy, short-term demand sensing using pattern recognitions, anomaly detections in assets, and inventory optimization, to name a few.
Today, AI is used in a wide range of applications, including image and speech recognition, natural language processing, and autonomous vehicles. More recent breakthroughs, such as Dall-E2 and ChatGPT from OpenAI, are rapidly opening new doors, as evidenced by our recent launch of Dynamics 365 Copilot. However, most companies are still focused on analytics and promotion use cases, such as forecasting demand or planning production.
As such, organizations have yet to fully explore the potential of AI, which involves self-learning supply chains, more sophisticated supply chain algorithms, and recognizing patterns in big data that are beyond human perception. AI can automate many of the recurring decisions in SCM and interact with supply chain systems in human context, but this requires a platform to connect legacy and modern solutions to unify the vast, growing amounts of supply chain data.3
Microsoft Supply Chain Platform and next-generation AI
As supply chain complexity grows, companies are using next-generation AI to gain a competitive edge and remain profitable. AI is proving to be a game-changer for businesses, whether they’ve already embarked on a digital transformation journey or are considering doing so. Let’s explore some of the cutting-edge AI use cases in supply chain management that can deliver immediate value without undertaking costly transformation initiatives.
AI-powered risk mitigation
By unifying data sources and business applications and combining them with next-generation AI, companies can better predict and act on disruptions across channels, suppliers, and geographies. For example, the AI-powered Microsoft Supply Chain Center news module proactively flags external issues such as weather, financial, and geopolitical news that may impact key supply chain processes. Plus, predictive insights surface affected orders across materials, inventory, carriers, distribution networks, and more.
With Dynamics 365 Copilot capabilities, users can quickly turn these insights into action with contextual email outreach. With a custom and contextual reply, supply chain users can save time and collaborate with impacted suppliers to quickly identify new ETAs and reroute a purchase order (PO) based on a weather disruption or fulfill a high-priority customer order via an alternate distribution center due to geopolitical tension.
Sign up for a free trial of Microsoft Supply Chain Center today.
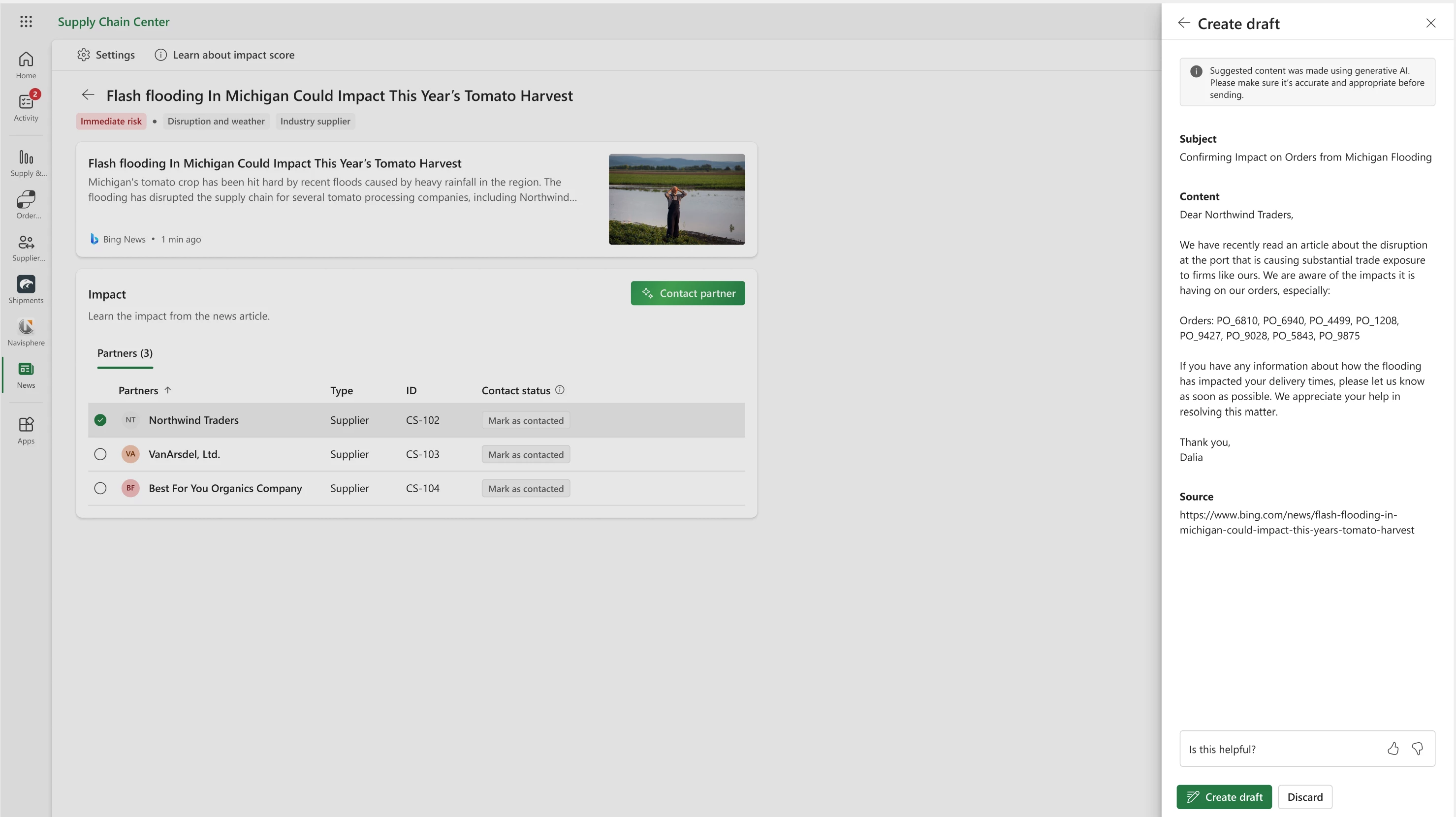
Optimize order fulfillment processes
Microsoft Dynamics 365 Intelligent Order Management (IOM) enables organizations to intelligently orchestrate fulfillment and automate it with a rule-based system using real-time omnichannel inventory data, AI, and machine learning. IOM can improve order fulfillment models by using AI to automate the identification and selection of optimized fulfillment decisions. Including the ability to enhance AI models when recommendations are not ideal, using the train, feedback, and improve methodology.
Elevate forecast accuracy with AI-driven collaborative demand planning
Demand forecasting is an area that has already seen pervasive use of AI. Organizations already use machine learning-powered forecasting algorithms to improve their forecast accuracy. However, trust in the system-generated forecast is still not as high as was hoped. Recent supply chain disruptions have only exacerbated the role of the importance of manual oversight during creation and careful review. As a result, demand planners and other stakeholders continue to spend a significant portion of their time manually analyzing trends and anomalies, and fine-tuning demand plans. The next-generation of foundation models have the potential to disrupt these very use cases. Ability to get answers through AI forecast explainability and natural language querying will help demand planners breeze through their demand plan analysis, reducing the time needed for fine-tuning and adjusting demand plan from days to minutes. Furthermore, AI can help in demand review meetings by using natural language for data-driven decision making, surfacing risks and opportunities, summarizing assumptions behind a plan, providing real-time what-if analysis, and generating transcripts and summaries of the meeting along with action items. The next generation of AI in demand planning promises to make the entire process more efficient, accurate, and collaborative.
Mitigate order delivery risks with data Q&A
Procurement teams often conduct monthly supplier reviews for top vendors by volume and vendors struggling to meet delivery requirements, but which have been painful to stop trading with for some reason. A significant amount of time for two to three team members is usually dedicated to gathering and analyzing monthly performance data in preparation for these reviews. Conversational AI can unlock productivity.
With conversational AI, we can imagine a future scenario where any analyst is prompting Dynamics 365 Copilot to: “Show me all orders which were not delivered on-time and in-full (OTIF) in the last 30 days. Estimate how much of our order backlog is impacted by these late deliveries. Suggest three questions that will help the supplier dig into the root cause of the issue. Write a short recommendation requesting the supplier participate in our monthly supplier review until OTIF is above 97 percent.” This example is only the tip of the iceberg for scenarios where generative AI can be used to democratize access and retrieval of a company’s data through conversation-styled interaction with AI chatbots.
Additionally, AI could significantly accelerate the onboarding of new suppliers by bypassing or speeding up internal legal review. We can envision purchasing managers, supply chain directors, and more benefitting from AI contract review by assisting in tasks like reviewing master supplier agreements.
Autonomous self-regulated supply chains
One of the biggest challenges in managing a complex supply chain is that it is “high touch” with disparate data sources, different cross-functional units, and processes ranging from strategy to execution. Companies struggle to harmonize these disparate data and processes, leaving planners to make intuition-based decisions rather than data-driven ones. AI can address the complexities of mapping a multi-tier network model from several disconnected systems across the value chain, including external business partners. Further, with advances in AI such as reinforcement learning, the networks can be adaptive, and self-regulated with different sub-network agents operating toward a common goal of increasing resilience, profitability, and customer service. Such an adaptive network considers the historical trends, and supply chain internal and external events, along with signals. The system evaluates multiple scenarios and performs business impact analysis to determine the best course of action using techniques such as simulation, optimization, and machine learning. For example, the system may recommend a make-and-buy option, versus solely buying, and provide a balanced scorecard of supply chain metrics and costs, along with a ranking for the recommendation. This type of supply chain offers automation to eliminate manual processes, and intervenes based on exceptions, generating alerts, and providing suggested actions. It also has the ability to self-learn from user actions and automatically execute corrective measures.
Intelligent process automation
As next-generation AI innovation emerges, it will increasingly deliver on the promise of automating many of the recurring decision touchpoints in supply chain management, freeing up valuable human resources to focus on higher-level productive tasks that require creativity, judgment, and complex problem-solving skills. AI bots can carry out tasks like reading email for new procurement requests, logging into multiple systems for data entry, solving supply chain alerts, and triggering workflows. Another example is increasing planners’ productivity by using generative AI to create the artifacts (plans, performance, assumptions, risks, and mitigations) required to run monthly business review (MBR) meetings such as sales and operations planning (S&OP).
Intelligent inventory visibility and optimization
Another example of AI in supply chain management is inventory intelligence where AI can balance inventory more accurately to reduce stockouts, and improve customer satisfaction and loyalty. Consider a scenario where the global inventory position analysis shows a projected inventory depletion in the upcoming quarter, with levels falling below safety stock requirements. With AI, the supply chain analyst can gain insights into the root cause—low supply relative to demand for a particular region and time. The scheduled maintenance of a factory in that quarter would lead to no additional production, exacerbating the situation. As a result, the demand must be met from existing inventory. With the help of AI-powered insights, the analyst can now delve deeper into the impacted products and locations and take corrective measures, using AI-powered recommendations to rebalance inventory from other locations or employing a cost-effective contract manufacturer.
Intelligent inventory visibility is also revolutionizing the way businesses search and view their stocks and products, empowering users with unparalleled accessibility and efficiency. The power of AI enables users to swiftly ascertain stock levels and product availability by merely typing their inquiries in natural language, similar to chatting with a friend. Whether it’s a query about products nearing expiration or the availability of limited-edition items across various regions, the AI assistant promptly delivers the desired results. Gone are the days of navigating through cumbersome menus, remembering product IDs or location details; simply use natural language to acquire essential information within seconds. In addition, AI can streamline today’s labor-intensive data-mining and table-joining. AI technology can now streamline the entire process, and even summarize inventory status in dashboard and text reports. Consequently, businesses can liberate their analysts from mundane tasks such as data cleansing and report writing, allowing them to focus on more strategic initiatives that drive success.
Shorten warehouse inventory cycle times
Another area of supply chain management to which AI can be applied is to shorten cycle times in warehouse fulfillment. Today, as demand for different items ebbs and flows, it’s difficult to predict which items should consume forward picking locations in the warehouse. Warehouses typically deal with the situation in two ways: they can pay for more space than they need at the current volume (unlikely) or have workers re-slot bins to bring items from a bulk area to a pick location. The latter is an ongoing, labor-intensive, and time-consuming process that is reactive by default.
In the future, AI could be applied to analyze incoming orders (or look further upstream in the supply chain) to forecast demand better. Based on this analysis, combined with data like physical product dimensions and the storage capacity of bin locations, recommendations for re-slotting can be offered to warehouse managers––allowing plans to be proactively set in motion to ensure that on-hand inventory is available at the time of picking.
Revolutionizing the supply chain industry
The above are just a few examples of how we imagine this next wave of AI innovation can improve supply chain processes and the overall employee experience. And it’s certainly just the starting point. AI has the potential to revolutionize supply chains, offering new possibilities for improved efficiency, cost savings, and customer satisfaction. To fully harness the benefits of AI, businesses must invest in the right technology and infrastructure to unify their supply chain processes and data—while considering critical aspects like security, accessibility, and company values. Microsoft is uniquely positioned to deliver AI in supply chain, by integrating built-in capabilities across our solutions and delivering a secure, composable, extendable, and interoperable platform. With low-code/no-code automation, collaborative actions, process orchestration, and rich supply chain functional capabilities in a single experience, customers can compose a tailored ecosystem and confidently apply AI to deliver new value.
Next steps for AI in supply chain management
Dynamics 365 Copilot in Microsoft Supply Chain Center is already unlocking a new world of opportunity and redefining what’s possible when teams harness the power of AI. By embracing AI, organizations can gain a competitive advantage and stay ahead of the curve in an ever-changing business landscape. And as we explored through the possible supply chain scenarios covered in the last section, we are just getting started. Sign up for a free trial to get started.
End notes
1 Four Ways Leaders Can Empower People for How Work Gets Done
3 Boston Consulting Group , 2022. Why AI-Managed Supply Chains Have Fallen Short and How to Fix Them.